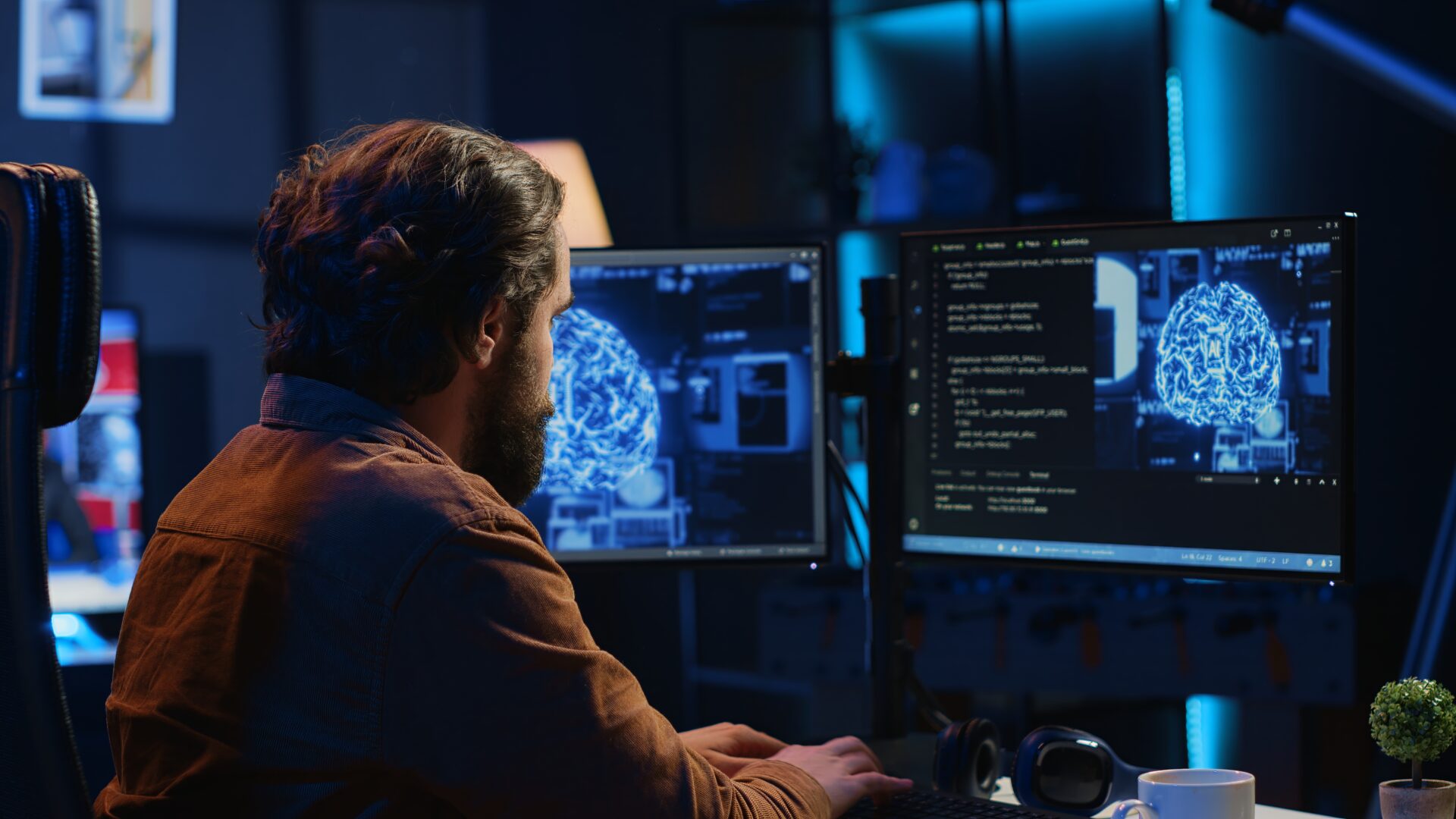
Introduction
In a knowledge-intensive global economy, organizations increasingly struggle to manage vast, distributed, and fast-growing bodies of information. While traditional Knowledge Management (KM) systems have helped address this challenge through documentation and structured repositories, they remain heavily dependent on manual inputs, resulting in inefficiencies, silos, and knowledge loss. With the advent of Artificial Intelligence (AI), KM systems can now automate tasks such as information retrieval, classification, and summarization. However, AI on its own lacks contextual judgment, ethical reasoning, and adaptability to complex, nuanced knowledge needs. Hybrid Knowledge Management (Hybrid KM) emerges as a transformative approach—merging AI’s speed and scalability with the strategic oversight and contextual expertise of human intelligence.
Overview of Hybrid KM
Definition and Concept of Hybrid KM
Hybrid KM refers to an integrated model where AI technologies such as natural language processing, machine learning, and generative AI are used to automate and optimize KM tasks, while human experts remain central to interpreting, validating, and contextualizing the outputs.
Hybrid KM differs from traditional or AI-only models by combining automation with human judgment, illustrated through the following features:
- AI performs data-intensive, repetitive, and large-scale knowledge tasks
- Humans ensure accuracy, contextual relevance, compliance, and trust
- The system continuously improves via feedback loops between machines and people
Hybrid KM is particularly valuable in environments where decision quality, data sensitivity, and regulatory compliance are critical.
Current Applications of Hybrid KM Across Industries
Industries that are both knowledge-rich and regulation-sensitive have been early adopters of Hybrid KM. These include:
The following use cases illustrate how Hybrid KM is operationalized in real-world settings.
Deep dive on Hybrid KM Use Cases
Healthcare – Enhancing Clinical Decision-Making
The exponential growth of medical knowledge creates a challenge for clinicians to stay updated while delivering high-quality patient care. Mayo Clinic addresses this through a Hybrid KM Program that combines AI with clinician oversight. Core solutions driving the approach at Mayo Clinics are:
Hybrid KM demonstrates how healthcare organizations can responsibly integrate AI into clinical practice while maintaining human control over quality, ethics, and patient safety.
Financial Services – Improving Secure Customer Support
In a sector where compliance and accuracy are paramount, AddAI.Life, an AI solutions provider, supported a financial services client in implementing a Hybrid KM system to transform customer support operations. Highlights of the implementation include:
Hybrid KM is enabling financial institutions to scale personalized support while meeting rigorous compliance standards—proving that AI and human oversight can work in harmony to elevate both service and security
Manufacturing – Accelerating Product Innovation
Manufacturers often struggle with data reuse, design consistency, and development timelines. Guangxi Yuchai Group, a leading engine manufacturer, applied Hybrid KM to unify product design, engineering, and production planning. Key Features of Yuchai’s Hybrid KM Framework include:
Guangxi Yuchai’s success highlights how Hybrid KM can drive digital transformation in manufacturing, enabling continuous innovation and operational agility across the product lifecycle
Consulting – Scaling Expertise with Hybrid KM
In consulting, where speed and accuracy in accessing acquired knowledge is critical, EY Global Delivery Services applies a Hybrid KM model that blends AI-powered platforms with human oversight to drive efficient, high-quality delivery. Key components of the approach include:
This integrated ecosystem embeds KM into daily consulting workflows, reducing manual effort and empowering professionals to focus on delivering high-impact client outcomes
Comparing Human-Only, AI-Only, and Hybrid KM
Hybrid KM bridges the gap between Human-Only and AI-Only KM systems in the following ways:
Implementation Roadmap for Hybrid KM
Organizations can implement Hybrid KM through a structured, six-phase roadmap that aligns technology, data, human expertise, and governance. This approach ensures a scalable, responsible, and context-aware KM system.
Phase 1: Establish Foundational Enablers
Before automation begins, organizations must build the core infrastructure that supports reliable and ethical AI-KM integration.
- Unified, Governed Data Infrastructure
– Use a data lakehouse1 to consolidate structured and unstructured sources
– Enforce data governance via access controls, metadata tagging, and lineage tracking
– Set up clean, normalized data pipelines2 across the enterprise
- Embedded AI Risk Controls and Policy Enforcement
– Integrate bias detection, drift monitoring, and content filters into AI workflows
– Automate policy compliance using configurable model governance tools
– Monitor outputs for Personally Identifiable Information (PII) leakage, hallucination, and toxicity
- Human Oversight for Context and Compliance
– Designate SMEs to review AI outputs and contextualize content
– Establish review boards for domain-specific oversight (e.g., clinical, legal)
– Embed human-in-the-loop controls in high-risk or regulated workflows
Phase 2: Automate Core KM Tasks with AI
– Deploy Natural Language Processing (NLP) tools to extract insights from unstructured documents
– Implement smart search, content classification, and auto-tagging to enhance knowledge discovery and accessibility
Phase 3: Add Human Oversight for Quality Control
– Assign SMEs to validate and contextualize AI-generated knowledge
– Apply explainable AI (XAI)3 principles to maintain transparency and build user trust
Phase 4: Enable Seamless Knowledge Sharing
– Centralize platforms that integrate AI-curated content with human-edited contributions
– Encourage collaborative knowledge sharing through forums, annotation tools, and community contributions
Phase 5: Embed Ethical and Regulatory Safeguards
– Implement AI filters to detect and mitigate bias, toxicity, and privacy risks
– Apply encryption and role-based access controls to secure sensitive knowledge assets
– Maintain audit trails for critical content to ensure traceability and accountability
– Align KM workflows with applicable laws along with sectoral standards
Phase 6: Track, Learn, and Improve
– Define measurable KPIs for usage, accuracy, and business impact
– Leverage feedback loops and performance dashboards to refine both AI systems and human processes iteratively
Ethical and Regulatory Considerations in Hybrid KM
Hybrid KM systems must be designed with ethical integrity and regulatory compliance in mind. These systems often operate across departments, data types, and jurisdictions—making it essential to embed safeguards that ensure trust, fairness, and legal adherence. Three key aspects of consideration are:
1. Responsible AI Design
Hybrid KM systems increasingly rely on AI for content discovery, recommendations, and automation. To maintain accountability and reduce harm, organizations must:
- Use explainable3 and auditable AI models, especially in decision-support use cases
- Apply filters to detect and prevent bias, toxic content, and personally identifiable information (PII) leakage
- Include human oversight, particularly in sensitive domains such as healthcare and finance, to ensure AI outputs are interpreted responsibly and ethically
These practices help align AI-driven knowledge processes with organizational values, regulatory expectations, and public trust
2. Multi-Level Legal Compliance
As organizations operate across sectors and geographies, Hybrid KM systems must comply with a layered regulatory environment. Importantly, compliance is determined not only by the location of the data subject, but also by the sensitivity and industry context of the knowledge being managed. This generally requires systems to align with regulations across three levels.
- Global frameworks, such as ISO 30401 (Knowledge Management Systems) and ITIL (IT service and delivery), offer universal guidance on structuring and governing knowledge effectively
- Sector-specific regulations introduce obligations tailored to industry contexts. For example:
– GDPR (EU) governs the protection and portability of personal data
– HIPAA (U.S.) sets strict standards for safeguarding health-related knowledge
– Basel Frameworks (global banking) defines compliance expectations for managing financial data and risk models - Regional and state-level regulations, such as the California Consumer Privacy Act (CCPA) and eIDAS (EU), add localized rules around data access, consent, and electronic verification
Hybrid KM systems should be designed to dynamically recognize and enforce the strictest applicable regulation based on user location, data category, and operational context
3. Governance and Risk Management
A robust governance structure ensures long-term compliance and adaptability. Organizations should:
- Maintain audit trails for knowledge creation, usage, and modification
- Enforce role-based access and encryption to protect sensitive content
- Conduct regular audits of AI performance and compliance status.
- Establish a cross-functional governance team to track regulation changes and guide system updates.
This governance layer ensures Hybrid KM systems remain compliant, trustworthy, and adaptable as regulations and technologies evolve
As the role of knowledge expands in shaping strategic decisions, embedding ethics and compliance into Hybrid KM is not just a risk safeguard—it’s a foundation for scalable, responsible, and future-ready organizations.
Conclusion: The Strategic Value of Hybrid KM
Hybrid Knowledge Management is the next frontier for organizations seeking to scale knowledge, improve decision-making, and build institutional intelligence. By combining AI’s automation capabilities with human insight and oversight, organizations can create KM systems that are faster, smarter, and more trustworthy.
From healthcare and banking to manufacturing and research, Hybrid KM is enabling enterprises to move from static knowledge repositories to dynamic, adaptive knowledge ecosystems—unlocking both efficiency and innovation.
References
- Forbes (2023)
- YourStory (2020)
- GDPR (2018)
- Mayo Clinic (n.d.)
- IBM (n.d.)
- Siemens (n.d.)
- ISO 30401:2018 (n.d.)
- CDC (n.d.)
- BIS (n.d.)
- State of California Department of Justice (n.d.)
- European Commission (n.d.)
Related articles
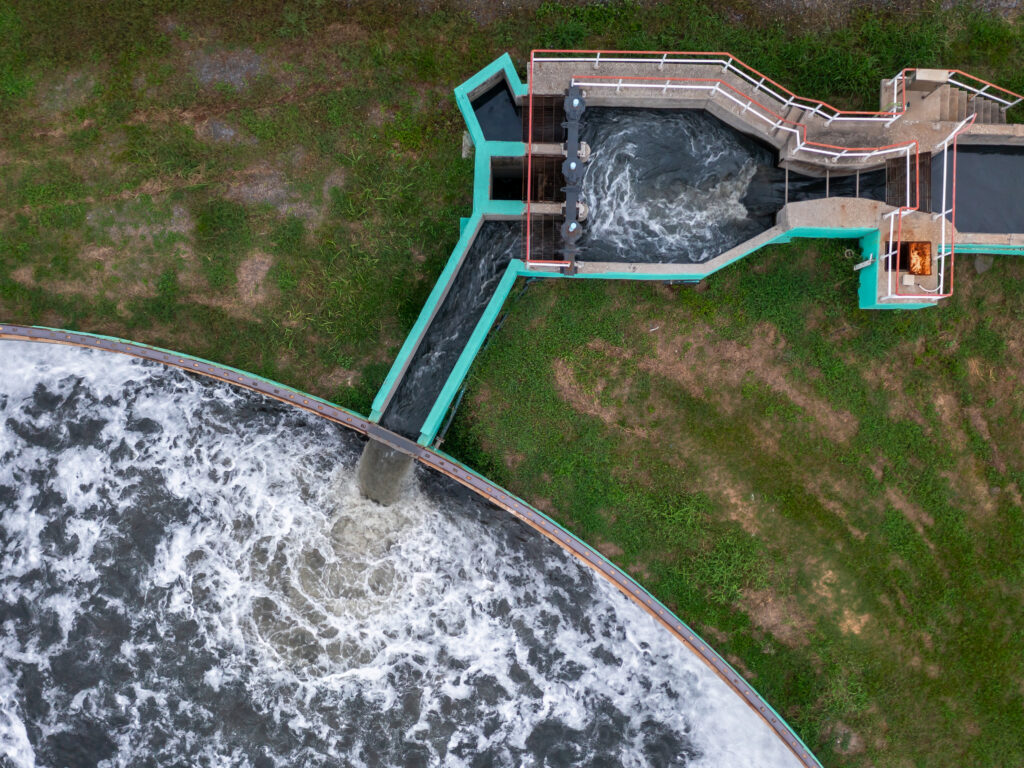
Rethinking Water Management for a Sustainable Future
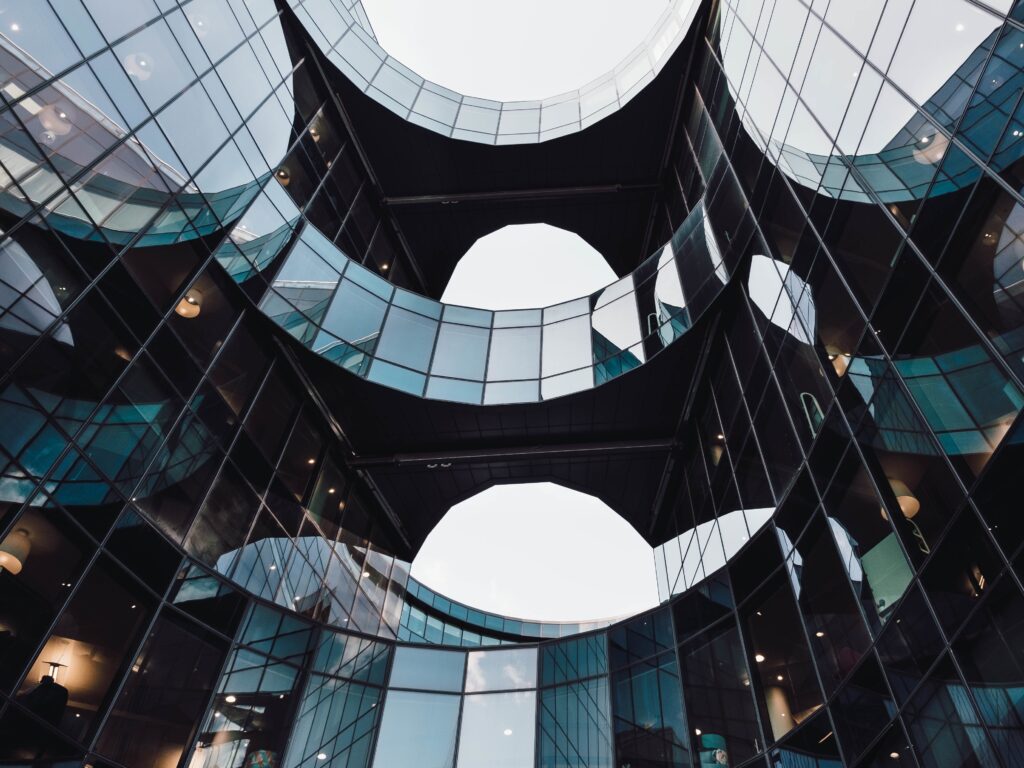
Leveraging Digital Twin Technology to Reshape the Customer Experience
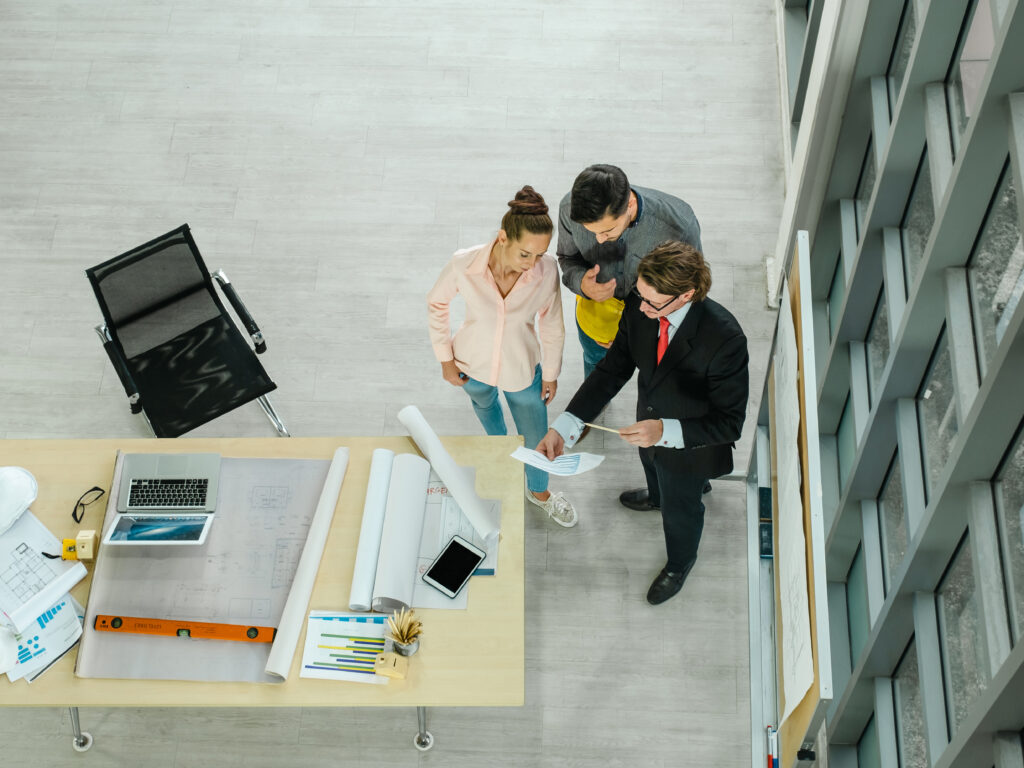